Professor Hideo Kuma from the Control Engineering Department of Matsue College investigates and aims to establish a new research goal by — 3D scanning deep inside narrow drift mines utilizing specially-developed remote-controlled robots. Additionally, Professor Kuma wants to establish a new method to estimate the start age and the production amount of drift mines across Japan by analyzing the ground surface around the entrances.
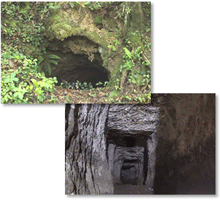
Entrance and drift of Iwami Silver Mine, a World Heritage
Obstacles to Investigating on Silver Mines of Historical Value
The remains of many mines are scattered across Japan including Iwami Ginzan Silver Mine which was certified as a UNESCO World Heritage Site in 2007 for its historical value of producing nearly one-third of the silver circulated around the world in the sixteenth century and later. Many mines are, however, now heavily wooded, and the entry is often restricted due to the risk of rock-fall. This makes it difficult, or even impossible to capture a complete picture and investigate these mines in detail. Therefore, initial drift mine research was mainly staged by recording the outside of drift mines through sketches and photos. Some 2D-scanning attempts were made inside some drift mines, but the obtained data was both inaccurate and insufficient for further investigations.
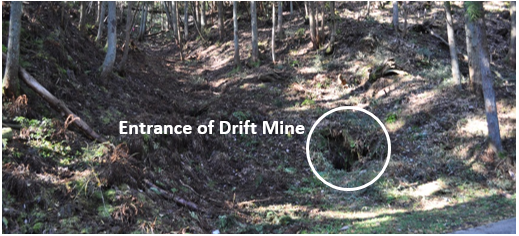
Heavily-wooded remain of drift mine
3D Scan the Ground Surface — Auto Detect and Remove Trees within the Point Cloud Data
One day at a trade show, Professor Kuma met with Elysium, the creators of point cloud handling software, InfiPoints. InfiPoints provides the functionality needed to extract the ground surface from the scanned point cloud data. He quickly introduced a new method to his research — 3D scan the remains of mines, and then extract the ground surface from the obtained point cloud data using InfiPoints.
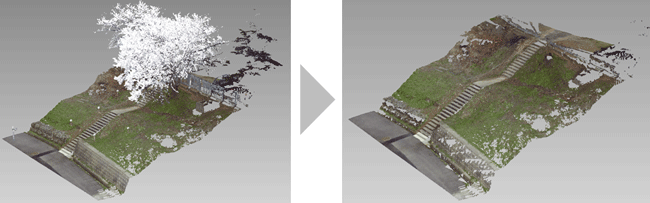
Extracting the points for ground surface only using InfiPoints
Professor Kuma began investigating using his new methods at Tada Silver-and-Copper Mine. Through this new method, he successfully identified the mark of open-pit mining, a characteristic surface mining technique.
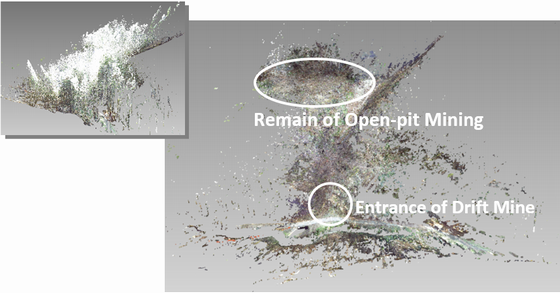
Point cloud data of Hyotan drift mine, Tada Silver-and-Copper Mine
Moreover, he was able to optimize the 3D scanned data for 3D printing using InfiPoints allowing him to create a model of the remains for further investigation. These 3D printed models allowed Professor Kuma to work on his second research goal of estimating the total amount of silver production based on the mark of open-pit mining.
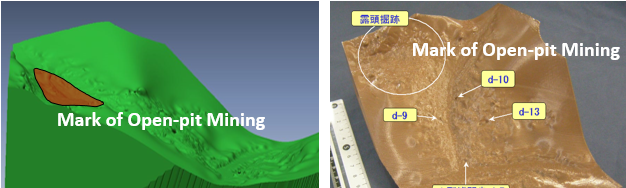
Left: Polygons generated from optimized point cloud data
Right: Model made by 3D printer
He even succeeded in unveiling the cultural landscape around the mine by minutely studying the ground surface and identifying the artifacts such as stone walls.
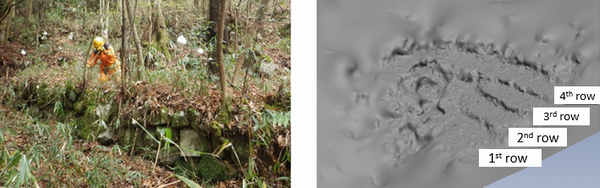
Left: On-site investigation of stone walls in Tada Silver-and-Copper Mine
Right: Polygon data of ground surface generated from 3D scanned data — stone walls came out clearly by removing points for woods in InfiPoints
Automatic Noise Reduction in the Scanned Data from Inside Drift Mines
The entry to the drift mine is often physically restricted, and even when it is possible, it is still very hard to conduct minute surveys for many hours inside the narrow, dusty drift mines. A special robot developed by students in Professor Kuma’s office has conducted unattended 3D scanning inside many drift mines. After preprocessing obtained point cloud data using InfiPoints — registering shorts, and removing noise caused by dust inside the drift —, the 3D scanned data can be fully utilized for their future virtual investigation and research.
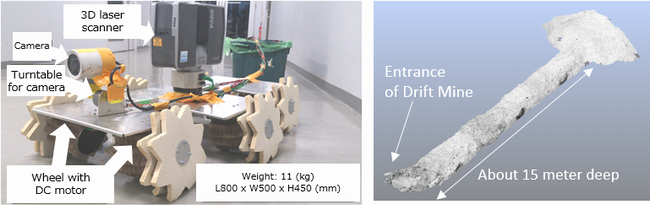
Left: Robot developed by Matsue College to carry 3D laser scanner and CCD camera
Right: Creating point cloud data of entire drift mine by registering 13 shots from 3D scanning
Possibility to Apply Point Cloud Handling Technology to Disaster Prevention
Professor Kuma has investigated about one hundred drift mine remains all over Japan, and he is seeing a great possibility to apply this 3D scanning and point cloud handling technology to disaster prevention. For example, detecting a risk of landslides through regular observations of mountain slopes and detecting variations.